Comparing impact of open-flow firewall on the SG-IoT-AMI-infrastructure, a well-designed cloud-based network against non-open flow firewall
Keywords:
Put Advance Metering Infrastructure, Computational Pipeline, Neural Network, Smart Grid, support vector machineAbstract
This work presents a novel smart grid tampering detection system re-engineered for end user monitoring and pipeline automation. The research focused on distributed energy resources. In context, the end user load profile, and generation capacity were processed in the cloud environment for tampering management. Computational pipelined methodology was adopted using baseline data from an independent electricity consumption data from 2018-2021 Abuja. First, a smart grid (SG) survey was carried using existing home estate at Abuja to ascertain tampering procedures in distributed energy resource domain. From the energy survey, the system architecture was developed and implemented based on computational model curve for dynamic attack vector mitigation. An Unsupervised layered SG Architecture with local concentrator was introduced including Advanced Metering Infrastructure Smart Grid (AMI SG) gateway and control Load Balancer Docker Agent with Binomial sink.SG AMI Network packet processing Scheme and docker Orchestration were characterized for SG AMI Traffic Model. Furthermore, Smart Grid Internet of Things Coordinating Infrastructure (SG-IoT-CI) Dynamic Resource Allocation and Load Balancing/Scheduling was presented. The results showed that there was a significant improvement when leveraging SG-IoT-CI unsupervised grid management. Also, a robust distributed SG-IoT based management architecture that links the processes for end-users was developed. To determine the efficiency of the computational algorithm for SG grid deployment, an experiment was carried out on SG-IoT-CI-AMI optimization model using schemes such as K-Nearest Neighbourhood with Isolated Forest (KNN +IF), Load Prediction with Regression (LPBSVR), Support vector machine (SVM), Load Prediction with Neural Network (LPBNN), Local Outlier Factor (LOF) and Lightweight On-line Detector of Anomaly (LODA) for validation study. For Full Scale Query Response Time (FSQRT) under Open Flow security control, it was observed that the SG-IoT-CI AMI Overflow and Non-Open Flow gave 47.82% and 52.17% respectively from the simulation statistic engine. Full Scale Resource Utilization under Open Flow security aggregation layer and Non Open Flow security aggregation layer gave 2.73% and 92.27% percentile utilization respectively. Using the unsupervised contexts, the Secure SG-IoTCI_AMI Latency for LPBSVR, Proposed KNN +IF, SVM, LPBNN, LOF and LODA gave 20.96%, 11.98%, 19.31%, 19.76%, 19.01% and 8.98% respectively. Secure SGIoTCI_AMI service rate gave 14.03%, 35.09%, 5.26%, 8.77%, 15.79% and 21.05% respectively. Secure SG-IoTCI_AMI Throughput gave 19.13%, 25.04%, 19.27%, 15.47%, 18.28% and 28.12% respectively. Secure SGIoTCI_AMI Accuracy Response gave 26.66%, 31.11%, 15.55%, 0.00%, 22.22% and 4.46% respectively. The results show that tampering control within SG grid ecosystems is feasible and very efficient.
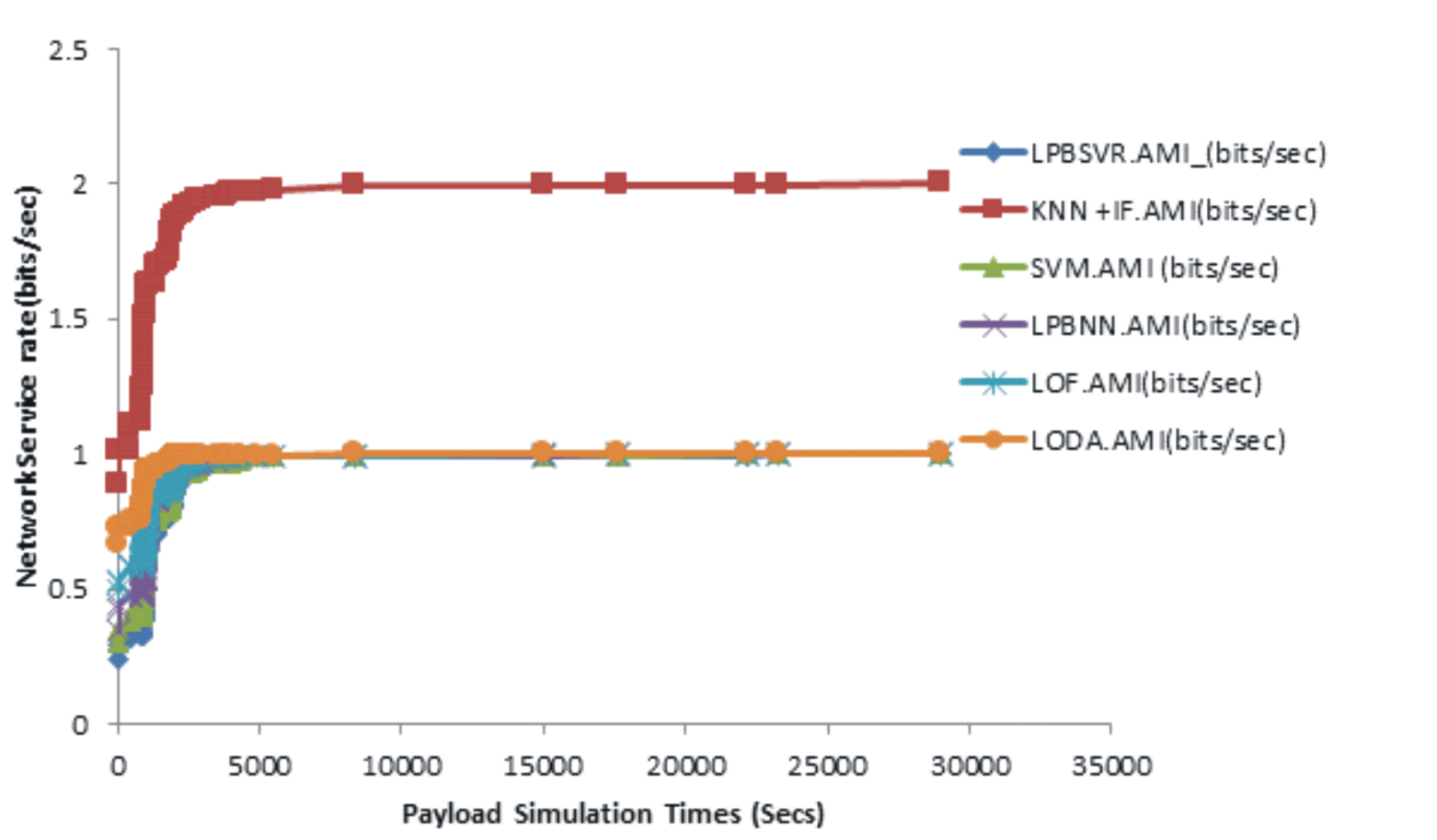