Monitoring of Migration of Harmful Sparrow Birds in a Rice Farm Using Convolutional Neural Network (CNN) Model
Keywords:
Convolutional Neural Network, Migration, Harmful Sparrow Bird, Image Classification, Rice FarmAbstract
This paper presents the development of a trained convolutional neural network (CNN) model to monitor migration of harmful sparrow birds in a rice farm. 98% of grain eaters are sparrow birds and there is need to monitor their movements across rice farms so as to reduce losses. This study grouped rice birds into beneficial (insectivorous) birds that feed on rice insect pests and the harmful sparrow birds that feed on the rice grain. Here, the prevalent harmful sparrow birds were identified and the different images of the birds were captured by high resolution cameras to form part of the datasets for the training of the CNN model. To monitor the movement of different species of harmful sparrow birds in and out of a rice farm, a total of 85,000 images of sparrow birds (100 images of the sparrow birds captured from the selected rice farm and 84,900 images of different sparrow birds across the globe obtained from internet) were pre-processed to form datasets for the training of the CNN model in Google Colab platform using python programming. If the model identified a bird other than the sparrow birds, it recognized it as beneficial birds and allowed it to continue pest control in the farm. A bird repository database was developed along with the dataset which contained information about the different species of the sparrow birds. Then, an algorithm was developed to use the classification of the CNN model to match the bird repository database so as to get information about the specie and origin of any sparrow bird that entered the rice farm and sent such information to the farmer as short message service (SMS). It helped the farmer to know whether to apply more aggressive and consistent bird deterrence or early harvesting of the crop in the rice farm depending on the frequency and nature of the sparrow bird detected. This model had accuracy of 98% in the detection and classification of the sparrow birds and will be of importance to agricultural researchers studying bird migration across farms.
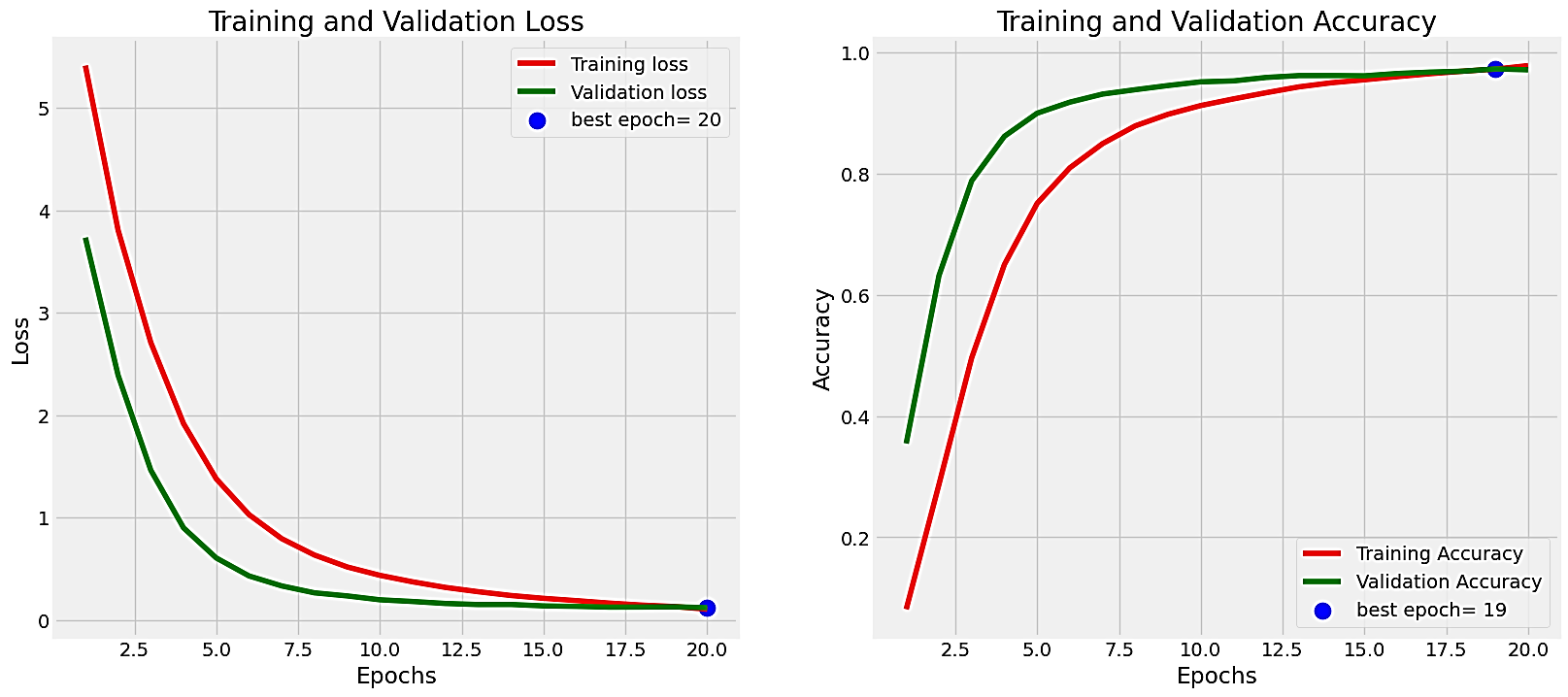