Coagulation performance of tiger nut chaff coagulant for treatment of fish pond wastewater: RSM and ANN machine learning Optimization
Keywords:
Coagulation, Tiger nut, Machine Learning, ANN, OptimizationAbstract
Chemical coagulants for wastewater treatment are sometimes expensive and environmentally hazardous, necessitating the search for eco-friendly alternatives. This study investigates the potential of Tiger nut chaff coagulant (TNCC) as a natural coagulant to treat fish pond wastewater FPW. The study focuses on optimizing the coagulation process with Response Surface Methodology and Artificial Neural Network (ANN) machine learning predictive analysis. The tiger nut chaff coagulant was extracted using a mixed salt solution procedure, and its coagulation efficiency was determined using the standard jar-testing method. The crude protein test conducted shows that TNCC contains 63.77% crude protein. The fish pond wastewater had an initial turbidity of 1213.9mg/L. After treatment, 91%, removal of the initial samples’ turbidity was observed. The maximum turbidity removal efficiency was a function of the temperature, coagulant dosage, pH levels, and concentration. Optimal turbidity removal was obtained at a pH of 6, 35 o C, 3 g of coagulant, and a concentration of 175 mg/L. The RSM- ANOVA lack of fit f-value of 0.2962 at the confidence level of 95% showed an insignificant lack of fit relative to pure error. The estimated R 2 and adjusted R 2 of the ANN model were 0.93023 and 0.91907, respectively showing high capability in capturing the nonlinear nature of the coagulation treatment of the Fish Pond effluent. The statistical R values (R > 0.94) obtained from RSM and ANN modeling of the coagulation treatment indicate their significant applicability in predicting experimental values. The average relative error within the model predictions and the actual outcomes was calculated using AARE. RSM produced a lower AARE deviation parameter of 0.02074 than ANN with AARE of 0.03583 which demonstrates higher prediction accuracy and superiority of RSM over ANN. The overall result suggests that TCN is effective as an alternative to chemical coagulants, furthermore, RSM provides a more robust optimal predictive function than ANN.
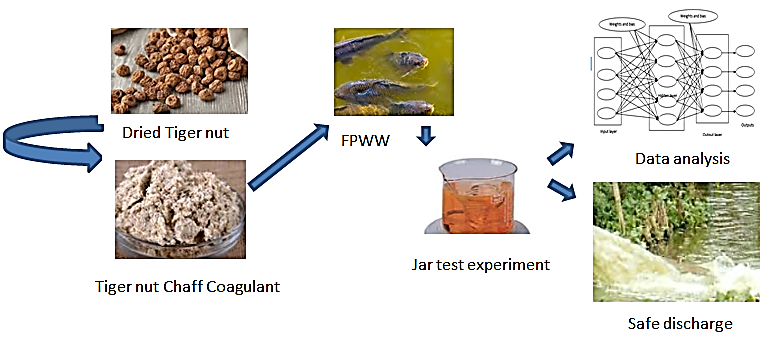