Optimized Power Loss Prediction in IEEE 118 Bus Network using Multilayered Feed-Forward Neural Networks
Keywords:
Neural Network, Neurons, Load flow, Levenberg-Marquardt, Newton RaphsonAbstract
Accurate power loss estimation is crucial for efficient power system operation and planning. Traditional methods rely on assumptions, leading to inaccuracies. This study employed Multilayered Feed-Forward Neural Networks (MFNNs) to develop a model that estimates real and reactive power losses in power lines. Load flow techniques were used to obtain variables for training several models. The desired model was selected after adjusting neuron numbers and comparing the performance indicators of other models. The 118-Bus IEEE test network was modelled using MATPOWER. The Levenberg-Marquardt backpropagation algorithm trained the model on generated data. Results show that the 25-neuron model performed best, achieving the least mean square error (0.00047543) at 1000 epochs. Correlation coefficients revealed a 0.99999 value for 20-neuron and 25-neuron models. The analysis identified the 25-neuron-based trained model as the most accurate for predicting power losses. It was observed that the 25-neuron model achieved optimum performance with the highest correlation coefficient (0.99999) recorded and the Least mean square error (0.00047543) at 1000 epochs. This study demonstrates the effectiveness of ANNs in estimating power losses in transmission lines. The recommended 25-neuron-based trained model provides the best predictions from studied models, enhancing power system efficiency and planning.
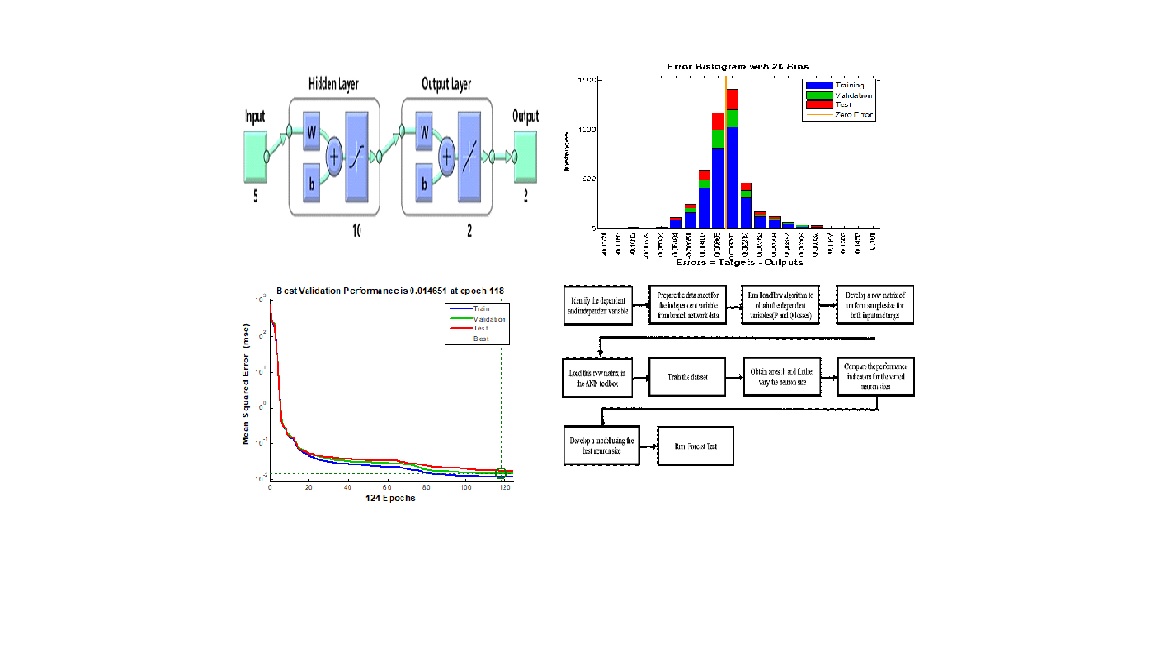