Leveraging Machine Learning and Data Analytics for Predictive Maintenance of Catalytic Converters for Optimal Emission Reduction Performances
Keywords:
Machine Learning, Data Analytics, Predictive Maintenance, Catalytic Converter, Emission Reduction and PerformancesAbstract
Catalytic Converters (CCs) play a crucial role in reducing harmful emissions and ensuring compliance with environmental regulations. Their efficiency impacts vehicle performance, operational costs, and emission standards. However, failures lead to increased pollution and maintenance expenses. This study addresses the challenge of optimizing CC maintenance using predictive maintenance (PdM) powered by machine learning (ML) and data analytics (DA). The objective is to develop ML-driven techniques to predict CC failures and optimize maintenance schedules. Key performance indicators (KPIs) such as exhaust gas composition, temperature, and pressure are monitored through embedded sensors. Collected data is analyzed using statistical methods like regression and clustering to model the relationships between KPIs and CC performance. Machine learning algorithms, including decision trees, random forests, and neural networks, predict degradation and failures. These models are trained on extensive datasets and validated with real-time inputs to enhance forecasting accuracy. Tools like MATLAB, Python, R, and Apache Spark facilitate statistical analysis and ML implementation, handling large-scale data efficiently. Results indicate that predictive models enable timely maintenance, reducing downtime and repair costs while enhancing CC lifespan and emission control. However, challenges include ensuring data accuracy, robustness, and system integration. Future work should focus on improving sensor reliability, refining hybrid modeling approaches, and enhancing real-time analytics to support sustainable automotive maintenance solutions.
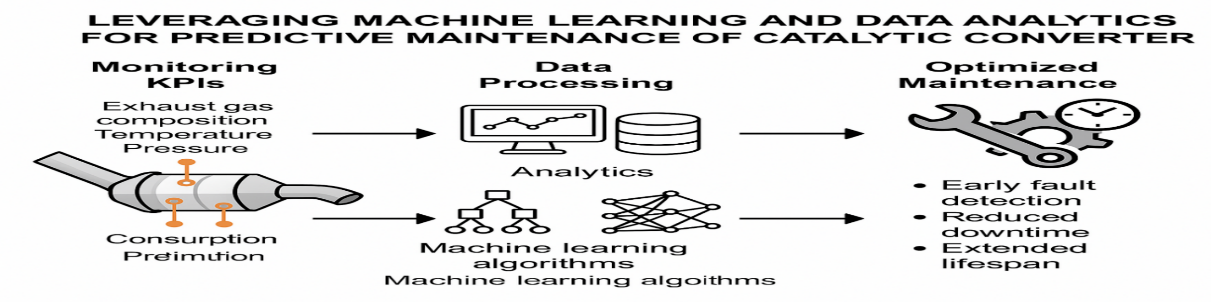