Comparative Analysis of Data Science Approaches for credit card Fraud Detection in the USA
Keywords:
Fraud detection, data science, credit card fraud, Logistic regression, Neural Network, Decision Tree, Random Forest classifier, Gradient Boosting modelAbstract
This study examined the usage of data science approaches to prevent fraud and financial loss in the credit card industry in United states of America. To achieve that, data on credit card fraud was collected from Kaggle which holds over 13000+ observation. The data was cleaned to ensure it usability and ability to fit models without overfitting. Six algorithms were used namely Logistic regression, Neural Network, Decision Tree, Random Forest classifier, Gradient Boosting model and Bagging model was used to identify the best model. However, all these models had accuracy above 0.93(93%) but we will choose Random Forest classifier as the best model with over 0.97(97%) accuracy, and it has the lowest execution speed which is the time needed for computation, data preprocessing, splitting and model evaluation. From the models, no of transaction, IP_address, average transaction and location are the main factor that affect the outcome of a transaction. Fraudsters spend a lot of time and resources looking for loopholes in models and credit card approval process so companies should be updated in various models they use and steadily look out for more opportunities for improving their transaction approval model.
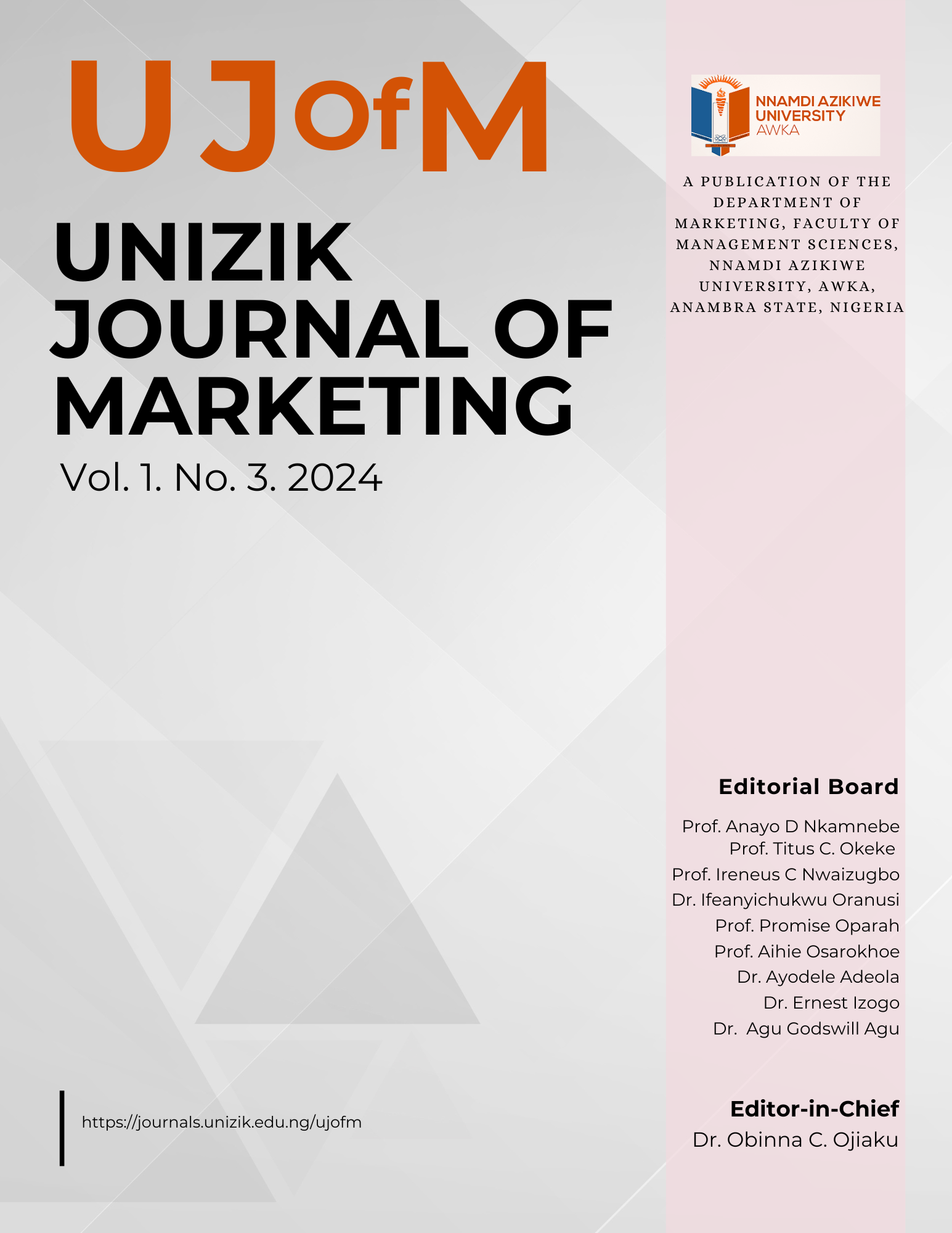
Downloads
Published
Issue
Section
License
Copyright (c) 2024 Ezenwafor, Ebuka Christian, Odezi, Jennifer Obuke, Onwujiobi, Charles

This work is licensed under a Creative Commons Attribution 4.0 International License.